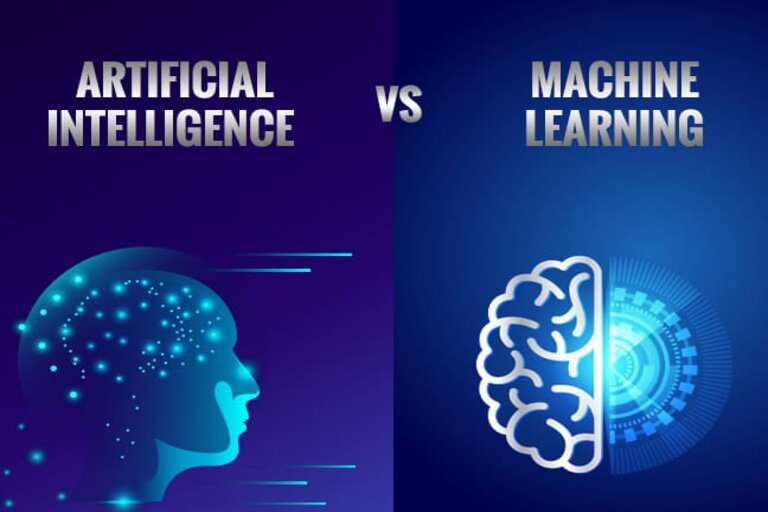
AI VS Machine Learning
Artificial intelligence (AI) and machine learning are intricately linked concepts in the realm of technology. When comparing AI and machine learning, it’s essential to understand their interconnected nature.
In the rapidly evolving landscape of technology, terms like Artificial Intelligence (AI) and Machine Learning (ML) are often used interchangeably or in conjunction with each other. However, they represent distinct concepts with specific applications and capabilities. Understanding the differences between AI and ML is crucial for grasping their respective roles in shaping our technological future.
Defining Artificial Intelligence (AI)
Understanding Machine Learning (ML)
Machine learning is a branch of artificial intelligence (AI) focused on using mathematical models to enable computers to learn and improve from experience without explicit programming. It allows computer systems to autonomously learn and make decisions based on data patterns.
One effective method of teaching a computer to emulate human reasoning is through neural networks. These are algorithms inspired by the human brain’s structure and function. Neural networks facilitate AI through a process known as deep learning, where the system learns complex patterns from vast amounts of data.
The distinction between AI and machine learning lies in their relationship: AI encompasses a broader range of technologies and goals, while machine learning serves as a specific approach within AI, emphasizing learning from data to enhance performance and decision-making capabilities. Therefore, the comparison of AI vs. machine learning underscores how these two fields complement each other in achieving intelligent systems.
Key Differences
Scope and Goals:
AI: Aims to create systems capable of simulating human intelligence across a wide range of tasks.
ML: Focuses on developing algorithms that can learn from data and improve performance over time without explicit programming.
Approach:
AI: Encompasses a broader set of techniques and methodologies, including symbolic reasoning, expert systems, and neural networks.
ML: Specifically focuses on statistical techniques and algorithms that enable computers to learn from data and make predictions or decisions.
Dependency on Data:
AI: Can utilize data for various purposes, including learning, reasoning, and decision-making, but not all AI systems depend on extensive datasets.
ML: Requires large amounts of data to train models effectively and improve accuracy over time.
Applications:
AI: Applied across diverse domains such as healthcare diagnostics, autonomous vehicles, virtual assistants, and gaming.
ML: Used in specific applications like recommendation systems, fraud detection, image recognition, and natural language processing.
Interconnections and Synergies
While AI and ML are distinct concepts, they are deeply interconnected:
ML in AI: Machine learning techniques often power AI applications, enabling systems to learn from data and perform intelligent tasks.
AI Beyond ML: AI encompasses broader capabilities beyond just machine learning, including reasoning, planning, and natural language understanding.
The Future Landscape
As AI and ML continue to advance, their impact on various industries and daily life is profound. From personalized healthcare to smart cities and autonomous vehicles, these technologies promise to transform how we live, work, and interact with the world.
Understanding the nuances between AI and machine learning is crucial for businesses, policymakers, and technologists aiming to harness their potential effectively. Both fields are driving innovation and reshaping industries, ushering in a future where intelligent systems enhance efficiency, creativity, and decision-making across the board.
while AI and machine learning are closely related, each plays a distinct role in advancing technology and driving progress. By comprehending their differences and synergies, we can better navigate the evolving landscape of intelligent systems and leverage their transformative potential responsibly and innovatively.