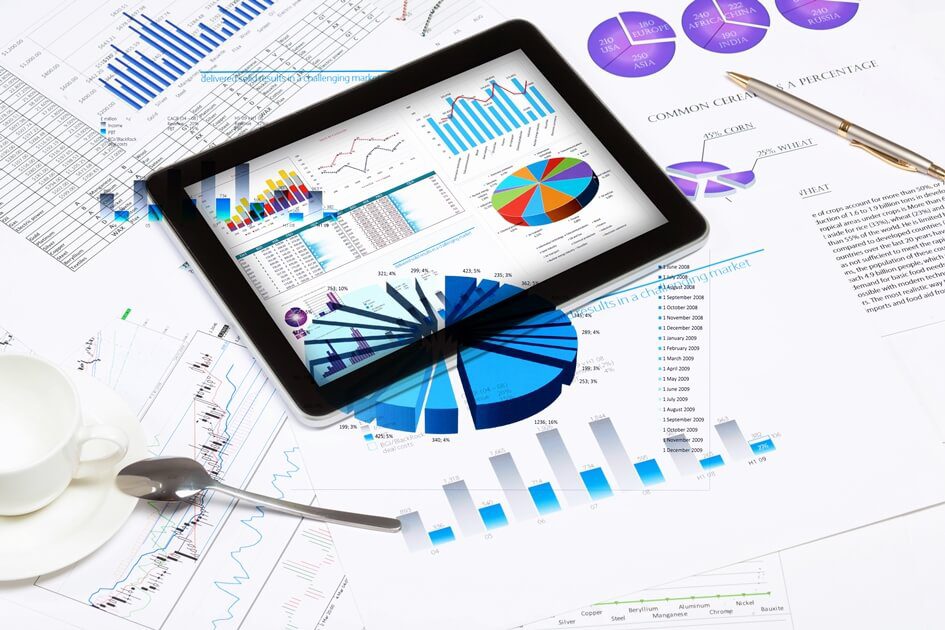
How to Analyze and Interpret Research
Analyzing and Interpreting Research is a process that involves looking at data collected through inquiry to figure out what the patterns may mean.
While data analysis and interpretation processes may be subject to pitfalls, they can still yield valuable insights. Understanding these pitfalls can help researchers and their teams to make informed decisions about the research they are conducting.
Analyze the Data
Data analysis involves inspecting, cleansing, and transforming the data collected in a way that uncovers useful information and supports decision-making. It can be performed with a variety of different tools, including descriptive statistics, inferential analysis, and quantitative analysis.
The first step in the data analysis process is to define your objective. This is a crucial step that will determine the type of data you need to collect and how you should present your findings.
Once you’ve figured out your objectives, it’s time to start collecting the data. But before you get started, it’s important to clean your data and make sure it’s error-free.
Develop a Hypothesis
Writing a hypothesis is a crucial step in any research project. It should be clear, concise, relevant and testable.
Hypothesis are statements about the relationship between independent and dependent variables. A simple hypothesis suggests only one variable, while a complex hypothesis indicates two or more independent variables and their relation to each other.
A hypothesis is an educated guess that comes based on knowledge, past studies, and/or a series of experiments. It is also grounded by valid facts.
A hypothesis should be able to explain the phenomena in question and provide evidence that will help you conduct future research. There are many ways to develop a hypothesis, but they all follow the same basic process: Start with a research question that is specific and focused.
Conduct a Reliability Test
A reliability test ensures that the data you collect is accurate. It also helps you to avoid biases that might have crept into your research, such as respondents’ changes in moods or environmental influences.
There are four types of reliability tests. Each method is used to measure different aspects of the same characteristic being measured.
Split-half reliability – This subtype of internal consistency reliability measures the correlation between two halves of items in a test that are designed to measure the same construct. You randomly split the items of the test into two sets and administer each set to a sample of respondents.
A correlation between the scores on the two sets is a good way to determine whether the items are similar. The higher the correlation, the more reliable the items are.
Conduct a Correlation Test
Correlation analysis is a quick and easy way to get a clearer understanding of how one variable affects another. It’s also a great tool for testing the effects of changing inputs while holding other variables constant.
To conduct a correlation test, start by importing the data into Excel. Once it’s done, click “Data” on the top bar menu.
Excel will then display the correlation coefficients of the correlated data. These are based on the Pearson and Spearman correlation coefficients.
Conduct a Regression Test
Regression analysis is an important statistical method used to analyze and interpret research data. It can be used to explain a phenomenon, predict things about the future, and identify risk factors that influence an outcome.
Linear regression is a common method for this task. It involves fitting a linear line through a cloud of data points to indicate the relationship between two independent variables and one dependent variable.
This method is often applied when a company wants to analyze a survey’s results and determine the impact of different factors on sales and revenue. It can also be used to estimate the effects of new products or promotions.
Conduct a Factor Analysis
Factor analysis uses a statistical approach to identify the interdependent relationships among different variables. Its goal is to reduce complex data sets to a few important dimensions.
Researchers use factor analysis in a variety of fields, including physiology, psychology, sociology and marketing. It can be used for theory development, psychometric instrument development and data reduction.
When conducting a factor analysis, it is important to choose which questions you want to analyze. Choosing the right questions is an art and a science, and it takes some experimentation, patience and creativity.
Once you have the data points you need, factor analysis produces loadings for each data point. These loadings describe how well the indicators correlate with a particular factor. Loadings range from -1 to +1, with stronger relationships being closer to -1 and weaker ones closer to zero.
Conduct a Regression Analysis
Regression analysis is an important tool for analyzing and interpreting research data. It can help you identify trends and potential opportunities for more accurate planning and decision-making.
Typically, regression analysis is used to determine the relationship between dependent and independent variables in order to make better business decisions. It also helps you avoid spurious correlations that can confuse your results.
Regression analysis can be done in a number of ways, including single linear and multiple regression. Simple linear regression uses a single dependent variable for each independent variable, while multiple regression uses multiple x variables for each independent variable.
Conduct a Multiple Regression Analysis
When you’re working with a data set that has many independent variables, you might want to do a multiple regression analysis. This will help you figure out how different factors affect a dependent variable, like sales.
When conducting a multiple regression analysis, you need to ensure that the model you create fits your data well. This is important because it will make sure that you get a valid result.
When a regression model is fit to your data, you will be able to check the model’s accuracy by looking at standardized residuals. This is important because it will show you whether your model meets the linearity, normality, and homogeneity of variance assumptions that you made when creating the model.
Conduct a Time Series Analysis
Time series analysis enables management to understand time-dependent patterns and trends in business metrics. For instance, it can be used for stock market price fluctuations or sales and turnover.
Time-series analysis can be analyzed into four main components: level, trend, seasonality, and noise. The first three are deterministic, while the fourth is non-deterministic.
The first part of the process is data cleansing, which removes outliers, applies averages, and filters out noise. This helps to gain a better overall view of the data and ensures that you are seeing the true signal of the data set.
Conduct a Cluster Analysis
Cluster analysis is a statistical technique that identifies and groups objects or data points according to their similarities. It also allows for analyzing how different segments of customers behave and interact with your company.
In marketing, clustering helps organizations discover distinct customer segments and develop targeted campaigns for each group. The purpose of clustering varies based on your organization and how it intends to use the results.
One common approach to clustering is k-means, which combines observations using a series of n-dimensional vectors that minimize Euclidean distances between them. The method is useful for finding clusters that contain missing data sets, but it should be used cautiously.